The Big Data Value Chain
Within the field of Business Management, Value Chains have been used as a decision support tool to model the chain of activities that an organisation performs in order to deliver a valuable product or service to the market (Porter 1985). The value chain categorizes the generic value-adding activities of an organization allowing them to be understood and optimised. A value chain is made up of a series of subsystems each with inputs, transformation processes, and outputs. (Rayport and Sviokla 1995) were one of the first to apply the value chain metaphor to information systems within their work on Virtual Value Chains. As an analytical tool, the value chain can be applied to information flows to understand the value-creation of data technology. In a Data Value Chain information flow is described as a series of steps needed to generate value and useful insights from data. The European Commission sees the data value chain as the “centre of the future knowledge economy, bringing the opportunities of the digital developments to the more traditional sectors (e.g. transport, financial services, health, manufacturing, retail)” (DG Connect 2013).
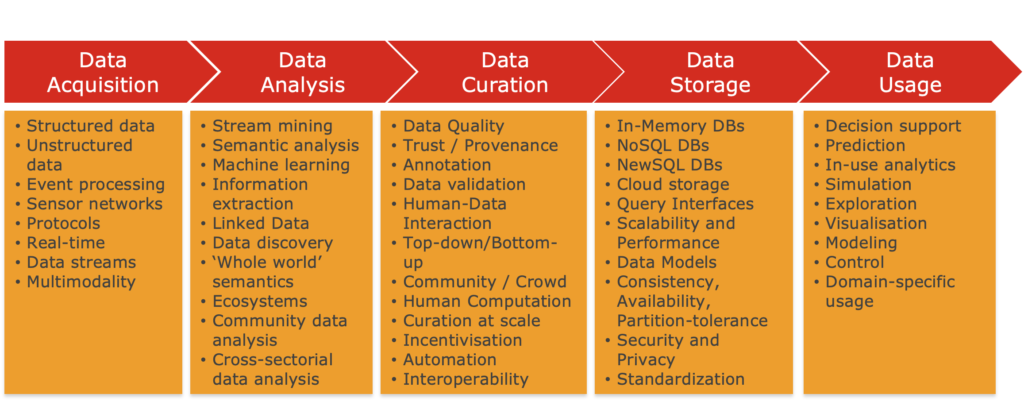
The Big Data Value Chain as described within (Curry E. et al. 2014)
The Big Data Value Chain (Curry E. et al. 2014), can be used to model the high-level activities that comprise an information system. The big data value chain identifies the following key high-level activities:
Data Acquisition: is the process of gathering, filtering, and cleaning data before it is put in a data warehouse or any other storage solution on which data analysis can be carried out. Data acquisition is one of the major big data challenges in terms of infrastructure requirements. The infrastructure required to support the acquisition of big data must deliver low, predictable latency in both capturing data and in executing queries; be able to handle very high transaction volumes, often in a distributed environment; and support flexible and dynamic data structures. Data acquisition is further detailed in Chapter 3.
Data Analysis: is concerned with making the raw data acquired amenable to use in decision-making as well as domain-specific usage. Data analysis involves exploring, transforming and modelling data with the goal of highlighting relevant data, synthesizing and extracting useful hidden information with high potential from a business point of view. Related areas include data mining, business intelligence, and machine learning. Chapter 4 covers data analysis.
Data Curation: is the active management of data over its life cycle to ensure it meets the necessary data quality requirements for its effective usage (Pennock 2007). Data curation processes can be categorized into different activities such as content creation, selection, classification, transformation, validation, and preservation. Data curation is performed by expert curators that are responsible for improving the accessibility and quality of data. Data curators (also known as scientific curators, or data annotators) hold the responsibility of ensuring that data are trustworthy, discoverable, accessible, reusable, and fit their purpose. A key trend for the curation of big data utilises community and crowdsourcing approaches (Curry et al 2010). Further analysis of data curation techniques for big data is provided in Chapter 5.
Data Storage: is the persistence and management of data in a scalable way that satisfies the needs of applications that require fast access to the data. Relational Database Management Systems (RDBMS) have been the main, and almost unique, solution to the storage paradigm for nearly 40 years. However, the ACID (Atomicity, Consistency, Isolation, and Durability) properties that guarantee database transactions lack flexibility with regard to schema changes and the performance and fault tolerance when data volumes and complexity grow, making them unsuitable for big data scenarios. NoSQL technologies have been designed with the scalability goal in mind and present a wide range of solutions based on alternative data models. A more detailed discussion of data storage is provided in Chapter 6.
Data Usage: covers the data-driven business activities that need access to data, its analysis, and the tools needed to integrate the data analysis within the business activity. Data usage in business decision-making can enhance competitiveness through reduction of costs, increased added value, or any other parameter that can be measured against existing performance criteria. Chapter 7 contains a detailed examination of data usage.
Excerpt from: Curry, E. (2016) ‘The Big Data Value Chain: Definitions, Concepts, and Theoretical Approaches’, in Cavanillas, J. M., Curry, E., and Wahlster, W. (eds) New Horizons for a Data-Driven Economy: A Roadmap for Usage and Exploitation of Big Data in Europe. Springer International Publishing. doi: 10.1007/978-3-319-21569-3_3.
References
- Curry E, Freitas A, O’Riáin S (2010) The Role of Community-Driven Data Curation for Enterprises. In: Wood D (ed) Link. Enterp. Data. Springer US, Boston, MA, pp 25–47
- DG Connect (2013) A European strategy on the data value chain.
- European Commission (2014) Towards a thriving data-driven economy, Communication from the commission to the European Parliament, the council, the European economic and social Committee and the committee of the regions. Brussels
- Pennock M (2007) Digital Curation: A Life-Cycle Approach to Managing and Preserving Usable Digital Information. Libr Arch J 1:1–3.
- Porter ME (1985) Competitive Advantage:Creating and sustaining superior performance. New York. doi: 10.1182/blood-2005-11-4354
- Rayport JF, Sviokla JJ (1995) Exploiting the Virtual Value Chain. Harv Bus Rev 73:75–85. doi: 10.1016/S0267-3649(00)88914-1
- Curry E, Freitas A, O’Riáin S (2010) The Role of Community-Driven Data Curation for Enterprises. In: Wood D (ed) Link. Enterp. Data. Springer US, Boston, MA, pp 25–47